Energy industry meets quantum machine learning
Quantum machine learning might play a pivotal role in the transformation of the energy industry. To combat climate change and reduce greenhouse gas emissions, renewable energy sources are being increasingly adopted. However, integrating these intermittent sources into existing power grids poses complex challenges. In this article, we elaborate on the potential of quantum machine learning, a fusion of quantum computing and advanced algorithms, to make a decisive impact on the energy sector for more sustainable solutions.
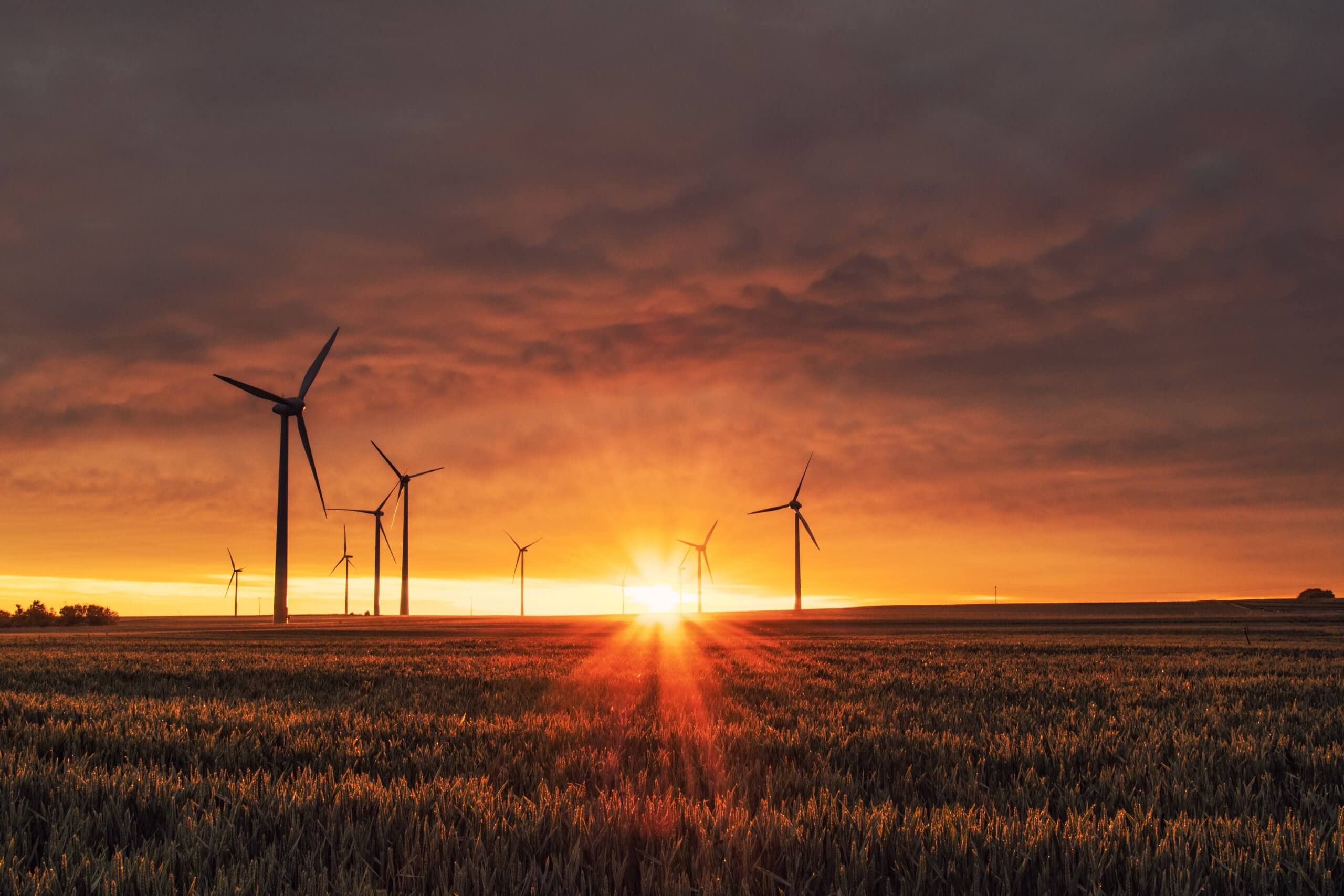
Quantum machine learning for optimized energy generation
Quantum machine learning can significantly impact the renewable energy sector. Traditional methods of predicting solar and wind power output have limitations in accuracy and scalability. Quantum machine learning algorithms, utilizing the computational power of quantum computers, have the potential to analyze vast amounts of data and identify patterns for more precise predictions. Accurate forecasts of renewable energy production could then enable grid operators to optimize power dispatch and integration, ensuring a reliable and stable supply of clean energy.
Quantum machine learning also aims to optimize the design and operation of renewable energy systems. By analyzing terrain data, weather patterns, and power generation potential, quantum algorithms can determine the optimal placement and configuration of solar panels and wind turbines. This maximizes energy output and efficiency, leading to cost-effective renewable energy installations.
Enhancing energy storage systems
The intermittent nature of renewables poses challenges to grid stability. Quantum machine learning can address this by optimizing energy storage systems. Quantum algorithms analyze historical energy production and consumption data to identify optimal strategies for energy storage, such as efficient charging and discharging times or power allocation for different time intervals. Optimized energy storage enhances grid stability, reduces reliance on fossil fuel backup power plants, and ensures efficient utilization of renewable energy resources.
Quantum machine learning could also contribute to the development of advanced materials to tackle the challenge of energy storage. By simulating and analyzing materials at the atomic level, quantum algorithms enable the discovery of novel materials with improved energy storage properties. This fosters the development of efficient and sustainable batteries, super-capacitors, and other energy storage technologies, facilitating the transition to a greener energy ecosystem.
QMware: Empowering greener energy with quantum machine learning
The potential of quantum machine learning for greener energy is immense. A challenge remains, as current quantum computers have limitations in computational power and stability. Therefore, QMware has dedicated its efforts to building a unique cloud platform leveraging the best of classical and quantum computing to unlock early benefits today. The QMware quantum cloud platform offers state-of-the-art quantum computing resources and a user-friendly interface for researchers, engineers, and businesses. By leveraging quantum algorithms and classical machine learning techniques, experts in energy optimization can unlock new insights, develop innovative solutions, and drive the adoption of sustainable energy technologies.
Explore our research papers
Get insights to QMware's hybrid quantum computing approach.
See publications